Distribute Code with Wheel Package
Pipeline execution in Databricks is done using predefined templates. These templates are python programs. Each template defines the data flow, for example - Data flow from RDBMS to AWS S3. This is accomplished using an RDBMS to AWS S3 template. The Calibo Accelerate platform provides several such templates out-of-the-box.
There can be different scenarios in which a new template needs to be deployed onto Databricks.
-
Scenario 1: When a new template is required, for example RDBMS to Azure Blob and such a template does not exist, then you need to create one.
-
Scenario 2: When there is a need to support a new feature or change an existing functionality, then an existing template undergoes changes.
In either cases, the new template has to be built as part of the wheel package and this wheel package needs to be updated on Databricks.
The following diagram depicts the process of invoking templates.
For changes to existing templates or creation of new templates, follow these steps:
Location of templates: plf-data-repository-util
Activity | Changes Required | Description |
---|---|---|
New template | Plf-data-repository-util | If there is a need for new template, then add new python template in the mentioned project. |
New logic in existing template | Identify the logic or technology to which the change belongs. Update the identified template (python) file. | Depends on the logic and technology. |
Build wheel package | Build new templates and prepare new version of wheel package. |
Deploy the wheel package to Databricks tool. In Jenkins pipeline, choose the environment to deploy. It will deploy the latest wheel package to the defined Databricks cluster. |
Deploy new version | Deploy new version of wheel package. |
Deploy the wheel package to Databricks. In Jenkins pipeline, chose the environment to deploy. It will deploy the latest wheel package to the defined Databricks cluster. |
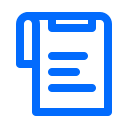
Note:For detailed instructions on building Jenkins pipeline and creating wheel package, refer to Jenkins and Python documentation.
Python Wheel Package
Introduction
This documentation provides insights into the Python wheel package functionalities, its integration with the Calibo Accelerate platform, and the overall workflow involved in enhancing and deploying features.
Lazsa Flow - Overview
The Calibo Accelerate platform empowers users with a user-friendly interface to design and execute complex data processing workflows. Users can leverage various nodes, such as AWS S3 and Databricks, to build custom pipelines for data transformation and analysis.
User Interaction and Workflow
The process begins with users dragging and dropping nodes within the Data Pipeline Studio user interface (UI). They configure parameters and initiate pipeline runs. The UI communicates with the backend Java services to trigger the pipeline execution. From there, the pipeline execution flows to the Databricks cluster.
Integration with Databricks
Once triggered, the pipeline execution is handed off to the Databricks cluster. Here, the power of Databricks is harnessed to process data efficiently. The Python wheel package plays a significant role in this process by enabling seamless integration with the cluster.
Generic Template and Process
We employ a generic Python notebook template within Java, facilitating interaction between UI and backend. This template calls the process_v2() method with all configured parameters from the UI. The template name drives the operation, invoking the appropriate method based on the template name.
Adding New features and Templates
To introduce new features or templates, the development process spans UI, backend services, and the Python wheel package. When introducing a new template, developers collaborate with UI and backend teams to define the template's name. Within the process_v2() method, the appropriate condition is set based on the template name. This ensures that the relevant method is called for the corresponding operation.
Development Workflow and Build Process
The process of incorporating new features involves multiple stages. Developers begin by parsing JSON from the UI to extract required parameters. A new file is created within the templates package to accommodate the new feature's code. After development is complete, the code is committed to the feature branch, and a pull request is raised for merging. Once approved, the code is built, verified, and versioned using our Jenkins build process.
Wheel Package Management and Deployment
-
Users have the flexibility to update the Python wheel package via the LASZA UI. In Data Pipeline Studio, for any pipeline that uses Databricks node a blinking dot near the ellipsis (...) on the UI indicates that an upgrade is available. Clicking on it opens a side drawer, allowing users to manage the wheel package. This streamlined process enables users to effortlessly upgrade or downgrade the package.
-
Users can install the Python wheel package using standard package management tools, such as pip on the cluster.
-
Or the user can install it from library section for the cluster.
Testing and Validation
Upon upgrading the wheel package, developers can execute pipelines with the new code to test changes. Rigorous testing ensures that the updated package functions seamlessly with the entire workflow.
Dependencies
-
List of all required dependencies mentioned in calibopythonutilssetup.py.
Notes
-
For the wheel version, we have specific files for all environments. For example dev-version.properties for dis environment.
What's next? RDBMS Adapters |